You may be confused as to how should data integrity and data quality be defined, so, let us look at some definitions first.
According to the “Clinical Trials Transformation Initiative” (CTTI), quality in clinical trials is defined as “the absence of errors that matter”.
Data professionals frequently define quality data as “data that is fit for purpose” (simply think at it as: “the data that is fit to answer the question asked by the study protocol”).
Data quality is defined by the regulators with objective and precise criteria. The GCP Inspectors Working Group from the EMA, in its “Reflection paper on expectations for electronic source data and data transcribed to electronic data collection tools in clinical trials – 9 June 2010 “, has defined the nine following attributes:
ALCOA : Attributable, Legible, Contemporaneous, Original, Accurate
+ CCEA : Complete, Consistent, Enduring, Available when needed
The ALCOA dimensions are one of the most critical focus for study monitors: they control that the source records are Attributable, Legible, Contemporaneous and Original when conducting the source data review (SDR), and they check also that the Case Report Form (CRF) records are Accurately representing the source records by conducting the source data verification (SDV). The dimensions of Completeness and Consistency are better controlled by data managers and statistical programmers from CRF data records in order to ensure that they meet the need of the study protocol and statistical analysis plan. They can use automated tools, which are much more efficient than on site monitoring visits to detect incomplete or inconsistent data. In addition, a cross-functional review of data can be used to spot inconsistencies which cannot be detected programmatically. The EA dimensions relate to the ability to retain the data records without alteration over time (“record keeping and archiving”).
The nine dimensions all together characterise the attributes against which data must be controlled, from source to reporting, in order to withstand a GCP inspection.
Data integrity can be easily understood as follows:
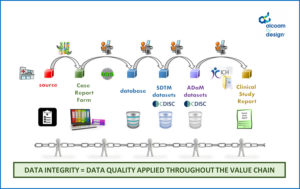
the chain that produces data is “only as strong as its weakest link”
Thus, to ensure data integrity and quality in an effective manner, a holistic approach is required, rather than an approach that reduces the production of quality to the sum of the quality control planned by each individual entity processing the data.
Imagine a situation where source data verification is planned to be conducted on 100% of data records by the monitors, whereas the transcription of a measure made from an image into a spreadsheet by a specialist central reader is left without quality control. What is the efficiency of spending travel costs and time from monitors to perform 100% SDV on site, if the central imaging parameter, used as part of the study primary efficacy endpoint evaluation, happens to be of poor quality?
Therefore, when trying to answer the question “do I feel confident that the integrity of my clinical study data is under good control?”, you should consider not only the quality delivered by the study sites and monitors, but also by the third party data providers (e.g. central labs), by the data integrators (e.g. the data managers who perform the integration of CRF and third party data to constitute the study database) and by the programmers of data transformations and reports (e.g. the statistical programmers producing the analysis datasets).
You should ask yourself:
-
- “who, within the sponsor or CRO team, has the 360° view on data integrity?”
-
- “who has the data management expertise required in order to be able to spot systemic weaknesses from the onset as well as during the study execution?”
-
- “is this person empowered to get the various data processing actors conduct the necessary preventive or corrective actions?”
Are you uncertain about the answer to the question now?
- “is this person empowered to get the various data processing actors conduct the necessary preventive or corrective actions?”
Or do you feel like, “yes, I believe I can be confident”, but would not answer “hand on heart”?
Perhaps a different perspective is needed then, which is to look at the objective evidence available to you in order to make you confident that the integrity of your clinical study data in under good control. This is what we are going to address in our next upcoming posts.
Isabelle Abousahl
President at alcoam by design SAS